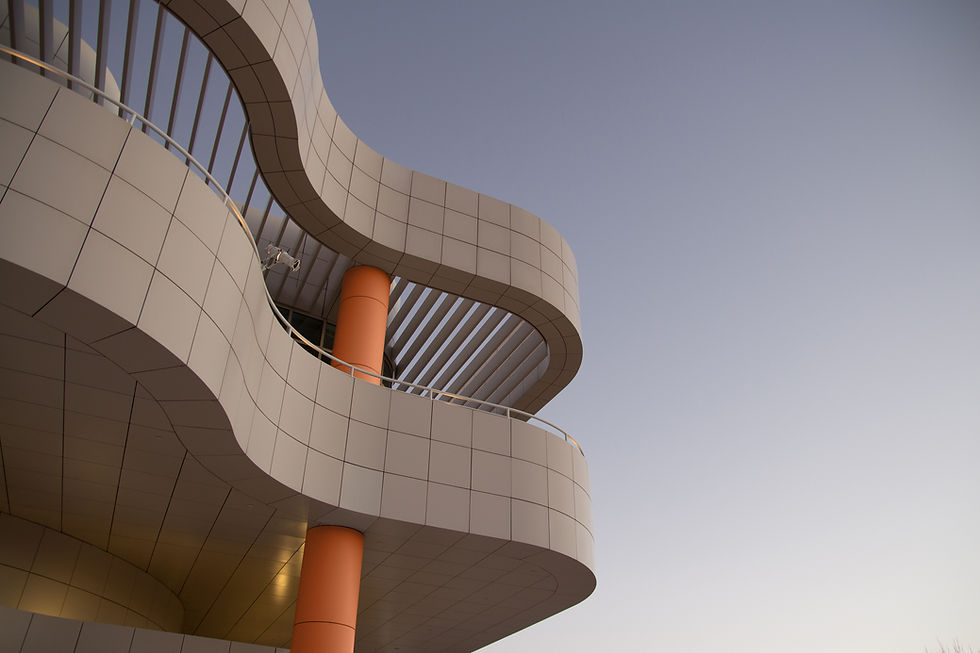

Introduction
Natural Language Processing (NLP) is a field of artificial intelligence (AI) that focuses on the interaction between computers and human language. It encompasses the development of algorithms and models that enable machines to understand, interpret, and generate human language in a way that is meaningful and contextually appropriate. NLP plays a crucial role in various applications, ranging from chatbots and virtual assistants to information retrieval systems and language translation services. By leveraging techniques from linguistics, statistics, and machine learning, NLP enables computers to process and analyze vast amounts of textual data, extract valuable insights, and communicate with humans in a manner that feels natural and human-like. As the scope of NLP continues to expand, it holds immense potential for revolutionizing industries, enhancing human-machine interaction, and transforming the way we process and utilize language.
In the following, I outline several applications of natural language processing that delineate the breadth of NLP's scope.
Directions of NLP
Text Generation and Language Generation
NLP facilitates the generation of human-like text through various techniques. Automatic summarization algorithms condense lengthy documents or articles into concise summaries, aiding in information retrieval and comprehension. Dialogue systems and chatbots employ advanced natural language generation techniques to engage in interactive conversations with users, providing assistance, answering questions, and simulating human-like conversation. Machine translation, another significant application of NLP, enables the automatic translation of text from one language to another, facilitating cross-lingual communication and content localization.
Language Understanding and Interpretation
Natural Language Processing (NLP) plays a pivotal role in extracting meaning and understanding from human language. Syntactic and semantic analysis techniques enable NLP systems to decipher the grammatical structure and capture the relationships between words and phrases. Sentiment analysis and opinion mining algorithms allow for the identification and interpretation of subjective information in text, providing valuable insights into public sentiment. Named entity recognition and entity linking are employed to identify and classify named entities, such as people, organizations, and locations, enabling information extraction and knowledge discovery from unstructured text data.
Information Extraction and Knowledge Discovery
One of the key objectives of NLP is to extract structured information from unstructured text data. Through entity extraction and relation extraction, NLP algorithms identify and classify entities and their relationships, enabling the creation of knowledge graphs and facilitating information retrieval. Event detection and sentiment analysis in social media help monitor and understand public opinion and sentiment trends. Text mining techniques enable the discovery of patterns, trends, and insights from large volumes of textual data, aiding in decision-making and data-driven research.
Question Answering and Conversational AI
NLP has made significant advancements in developing intelligent question answering systems. These systems analyze and interpret user queries, retrieve relevant information, and generate precise answers. Conversational agents and virtual assistants utilize NLP capabilities to engage in dynamic and context-aware conversations, providing personalized assistance and performing tasks on behalf of users. Chatbot technologies, powered by NLP, enable interactive and conversational interfaces for various applications, such as customer support, information retrieval, and entertainment.
Speech Recognition and Natural Language Understanding in Speech
The integration of NLP with automatic speech recognition (ASR) facilitates the transcription and analysis of spoken language. NLP techniques enable the conversion of audio inputs into text, enabling voice-controlled systems and applications. Voice assistants, such as Siri, Google Assistant, and Amazon Alexa, leverage NLP to understand user commands, answer questions, and perform tasks through voice interactions. Voice search, powered by NLP, allows users to retrieve information by speaking naturally, enhancing user experience and accessibility.
NLP in Healthcare and Biomedicine
NLP has found numerous applications in the healthcare and biomedicine domains. Medical text mining and clinical decision support systems utilize NLP techniques to extract valuable information from medical literature and electronic health records. NLP enables the extraction of medical knowledge, such as symptoms, diagnoses, and treatments, aiding in research, diagnosis, and patient care. Sentiment analysis of patient feedback and reviews helps assess the quality of healthcare services and identify areas for improvement. Disease classification and prediction models leverage NLP to analyze clinical narratives and identify patterns for early detection and treatment.
Applications and use cases of Natural Language Processing (NLP):
Customer Support and Chatbots
Information Retrieval
Sentiment Analysis
Machine Translation
Text Summarization
Question Answering Systems
Speech Recognition and Voice Assistants
Text Classification and Sentiment Analysis
Language Generation
Healthcare and Biomedicine
Legal and Compliance
Social Media Analysis
Named Entity Recognition
Document Classification
Text-to-Speech Conversion
Text Mining and Data Extraction
Fraud Detection
Opinion Mining and Review Analysis
News Analysis and Topic Modeling
Intent Detection and Dialogue Systems
Ethical Considerations and Bias in NLP
As NLP becomes increasingly pervasive, ethical considerations and biases in NLP systems have gained significant attention. Biases present in training data can lead to biased language generation or discriminatory outcomes. Ensuring fairness, transparency, and accountability in NLP applications is crucial. Efforts are being made to detect and mitigate biases, promote diversity in training data, and develop guidelines for responsible data collection and usage. Addressing ethical considerations and bias in NLP is essential to build inclusive and trustworthy systems.
Future Directions and Emerging Trends
The future of NLP is characterized by ongoing advancements and emerging trends. Deep learning and neural language models, such as transformer-based architectures, have revolutionized NLP, leading to improved performance in various tasks. Integration of NLP with other AI technologies, such as computer vision, enables multimodal analysis, where text is combined with images, videos, and other modalities. Explainability and interpretability in NLP models are gaining attention to enhance transparency and trust. Continued interdisciplinary collaboration, research, and innovation are key to shaping the future of NLP and unlocking its potential across industries and societal domains.
Conclusion
The scope of Natural Language Processing (NLP) is vast and continually expanding. From language understanding and interpretation to text generation, information extraction, question answering, and speech recognition, NLP has revolutionized how machines interact with human language. Its applications span across healthcare, customer service, information retrieval, and more. However, ethical considerations, bias mitigation, and ongoing research are essential to ensure responsible and inclusive deployment of NLP systems. With advancements in deep learning, multimodal analysis, and explainability, NLP is poised to further transform industries and enhance human-machine interaction in the future.